Three strategies to increase customer satisfaction in the contact center
Before Spotify grew to be the No. 2 music streaming platform in the U.S., many of its rivals simply copied its features, making it hard for Spotify to win repeat customers and long-term subscribers. To stand out, the company focused on better understanding its customers’ changing and growing needs by using the insights from its platform – insights that couldn’t be intuited by competitors – to develop new and better offerings.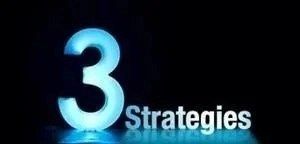
Pinpointing exactly how a business meets, surpasses or falls short of a customer’s expectations enables improvements and refinements that have a powerful impact on the bottom line. A study found that customers are willing to pay 16% more for better customer service, while bad customer service costs businesses up to $62 billion per year.
To increase customer satisfaction and define satisfaction rates, organizations must start with their contact center analytics. Agents play one of the most critical roles in customer-brand interactions, but by taking a closer look at contact center data, enterprises can also identify challenges impacting the brand-customer relationship in other areas of the business.
Tapping into this data requires powerful, artificial intelligence (AI)-driven analytics that can identify patterns, trends and anomalies affecting customer satisfaction. That’s because the volume of data and number of data sources required – from IVR, brick-and-mortar stores, billing, agent notes, calls, emails, voice, web and more – are difficult or time-consuming for employees to unify, prepare and analyze on their own.
To begin using analytics to identify and boost customer satisfaction rates, focus on implementing or adopting these three strategies.
Understand customer sentiment to increase satisfaction
One of the most sought-after indicators of customer satisfaction relates to the emotions that customers have during an interaction -- their sentiment. Understanding sentiment typically requires measuring whether customers have a positive, negative or neutral experience in a contact center interaction. Gathering and analyzing that data provides evidence needed to make change, identify the specific source of dissatisfaction and understand the nature of specific issues.Pinpointing customer sentiment is notoriously difficult to do at scale, as it requires analysis of spoken words, speech characteristics like pitch and tone, and situations where agents and customers are “cross-talking” or interrupting each other. That’s why many organizations are turning to artificial intelligence to detect sentiment and drive customer satisfaction.
AI-powered sentiment analysis models can help organizations understand:
- What words and phrases indicate positive or negative sentiment.
- Whether positive phrases offset negative ones.
- The tone used by customers and agents and how it impacts sentiment.
- Whether laughter is present, indicating positive sentiment.
- When cross-talk is happening and whether it indicates positive or negative emotions.
- The acoustic properties of agents’ and customers’ voices, like intensity or speaking rate, that reveal sentiment.
- The sentiment expressed by customers on specific topics and queries, and how frequently.
Single out the behaviors that enable rapid customer satisfaction improvements
Specific agent behaviors can have an outsized affect on whether a customer has a great interaction. The skills that can make or break a customer’s experience most frequently are soft skills, like how friendly an agent is and whether the agent actively listens to a customer, owns a customer’s issue and shows empathy. A Salesforce study shows just how important these behaviors can be: Customers ranked efficiency, ability to handle requests without transfers, empathy and politeness among the most important traits customer service representatives should have.These qualities, however, are inherently subjective and difficult to define – what one person considers friendly or empathetic can come across as rude or overbearing to another. Consider the case of a large enterprise company that was spending millions of dollars each year trying to improve soft skills. Its research and development department ran a test to see if agents could be objectively evaluated based on soft skills. The department had multiple supervisors listen to the same interactions and score each agent based on the soft skills used in the call – and there was no consensus among the supervisors.
Conflicting scores and insight from manual analysis of calls damages employee experience and obscures what agents need to be doing to improve. Manual analysis also requires listening to hundreds or thousands of individual calls, which is highly time-consuming and expensive from a staffing perspective. So how can businesses improve soft skills at scale?
This is where AI analytics’ predictive and interpretive models come in. AI removes the subjectivity inherent in manual soft skills analysis. It can automatically score 100% of interactions to determine whether agents are using critical soft skills, identify where they need to improve and provide specific and objective insights that supervisors can use for coaching.
Operationalize insights to strengthen customer satisfaction
Once enterprises have insights into what’s affecting customer satisfaction, it’s critical to drive change on a day-to-day basis within operations. Having the information on what to change is one thing, but making it happen at the functional, department, group or agent level requires an additional strategy.What organizations need is a next-generation quality management solution that operationalizes analytics findings, making it easy to effect real-world change based on data insights. Analytics-powered quality management enables businesses to connect customer needs to specific agent performance metrics.
Look for solutions that analyze and auto-score 100% of interactions and can link findings from analytics to agents’ behaviors. This provides the context needed for supervisors and coaches to educate agents about the specific behaviors and actions needed to improve customers’ experience. Solutions that make insights easily accessible with dashboards and automate evaluation of all interactions will effect the most change.
In today’s digitally driven world, customer satisfaction improvements cannot be achieved with guesswork, as Spotify found – whether an established brand or an upstart disrupting an industry, organizations need real-world, real-time insights drawn from a broad variety of sources that can be easily put into action. NiCE Enlighten AI makes it easy for organizations to begin increasing customer satisfaction in the contact center, either post-interaction or in real-time. Learn more by visiting our Customer Engagement Analytics solutions.Learn more about customer experience analytics >>